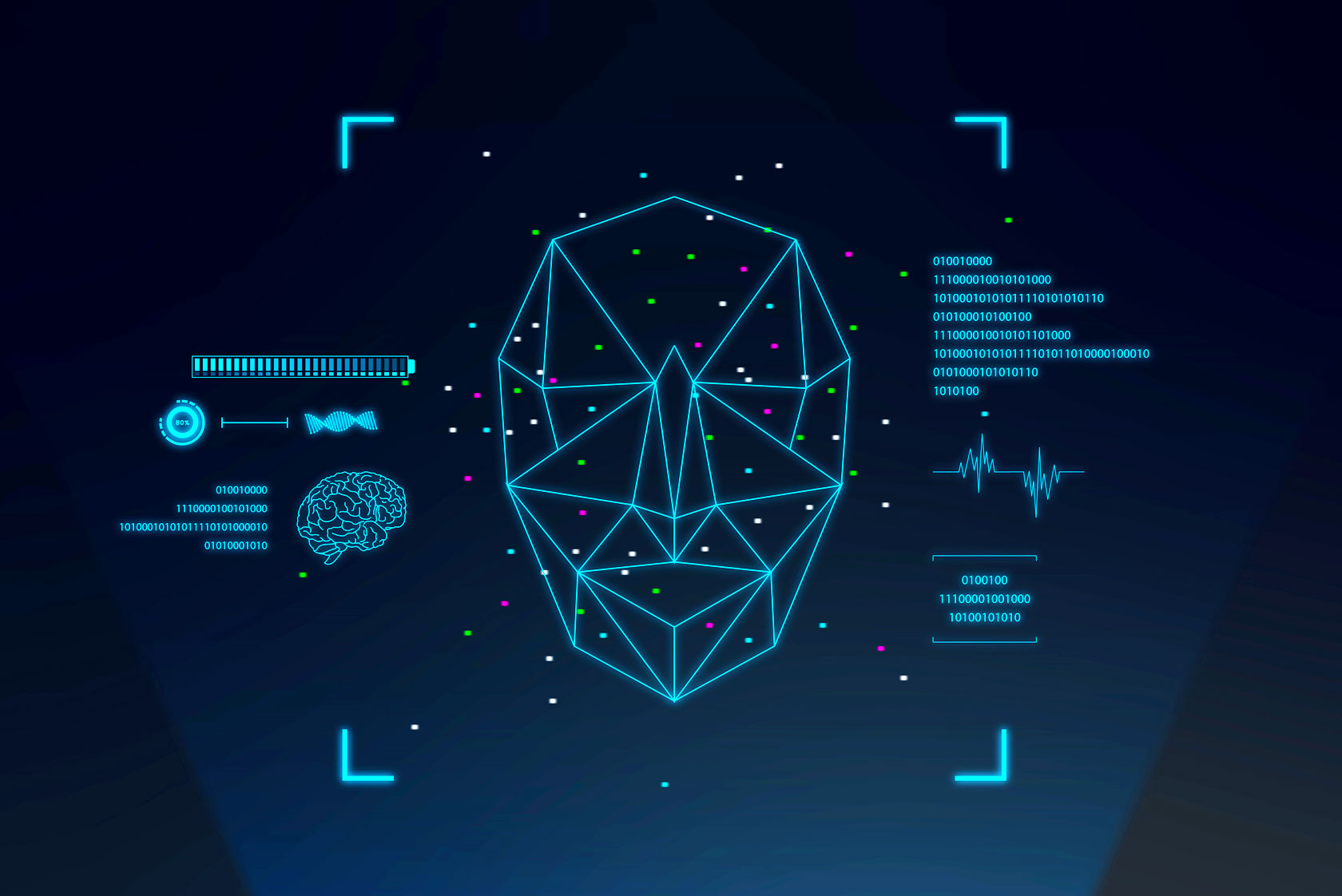
Discrimination Through AI: To What Extent Libraries are Affected and how Staff can Find the Right Mindset
Artificial intelligence (AI) has become an indispensable part of the modern scientific system. But who ensures that such AI systems do not discriminate against anyone and draw false conclusions from available data? And what do libraries have to do with it?
An interview with Gunay Kazimzade (Weizenbaum Institute for the Networked Society – The German Internet Institute)
Gunay, in your research, you deal with the discrimination through AI systems. What are typical examples of this?
Typically, biases occur in all forms of discrimination in our society, such as political, cultural, financial, or sexual. These are again manifested in the data sets collected and the structures and infrastructures around the data, technology, and society, and thus represent social standards and decision-making behaviour in particular data points. AI systems trained upon those data points show prejudices in various domains and applications.
For instance, facial recognition systems built upon biased data tend to discriminate against people of colour in several computer vision applications. According to research from MIT Media Lab, white male and black female accuracy differ dramatically in vision models. In 2018, Amazon “killed” its hiring system, which has started to eliminate female candidates for engineering and high-level positions. This outcome resulted from the company’s culture to prefer male candidates to females in those particular positions traditionally. These examples clarify that AI systems are not objective and are mapping human biases we have in society to the technological level.
How can library or digital infrastructure staff develop an awareness of this kind of discrimination? To what extent can they become active themselves?
Bias is an unavoidable consequence of situated decision-making. The decision of who and how classifies data, which data points are included in the system, is not new to libraries’ work. Libraries and archives are not just the data storage, processing, and access providers. They are critical infrastructures committed to making information available and discoverable yet with the desirable vision to eliminate discriminatory outcomes of those data points.
Imagine a situation where researchers approach the library asking for images to train a face recognition model. The quality and diversity of this data directly impact the results of the research and system developed upon those data. Diversity in images (Youtube) has been recently investigated in the “Gender shades” study by Joy Buolamwini from MIT Media Lab. The question here is: Could library staff identify demographic bias in the data sets before the Gender Shades study was published? Probably not.
The right mindset comes from awareness. Awareness is the social responsibility and self-determination framed with the critical library skills and subject specialization. Relying only on metadata would not be necessary for eliminating bias in data collections. Diversity in staffing and critical domain-specific skills and tools are crucial assets in analysing library system digitised collections. Training of library staffing, continuous training, and evaluation should be the primary strategy of the libraries on the way to detect, understand and mitigate biases in library information systems.
If you want to develop AI systems, algorithms, and designs that are non-discriminatory, the right mindset plays a significant role. What factors are essential for the right attitude? And how do you get it?
Whether it is a developer, user, provider, or another stakeholder, the right mindset starts with the
- Clear understanding of the technology use, capabilities as well as limitations;
- Diversity and inclusion in the team, asking the right questions at the right time;
- Considering team composition for the diversity of thought, background, and experiences;
- Understanding the task, stakeholders, and potential for errors and harm;
- Checking data sets: Consider data provenance. What is the data intended to represent?;
- Verifying the quality of the system through qualitative, experimental, survey, and other methods;
- Continual monitoring, including customer feedback;
- Having a plan to identify and respond to failures and harms as they occur;
Therefore, long-term strategy for library information systems management should include
- Transparency
- Transparent processes
- Explainability/interpretability for each worker/stakeholder
- Education
- Special Education/Training
- University Education
- Regulations
- Standards/Guidelines
- Quality Metrics
Everybody knows it: You choose a book from an online platform and get other suggestions a la “People who bought this book also bought XYZ”. Are such suggestion and recommendation systems, which can also exist in academic libraries, discriminatory? In what way? And how can we make them fairer?
Several research findings suggest making recommendations fairer and out of the “filter bubbles” created by technology deployers. In recommendations, transparency and explainability are among the main techniques for approaching this problem. Developers should consider the explainability of the suggestions made by the algorithms and make the recommendations justifiable for the user of the system. It should be transparent for the user based on which criteria this particular book recommendation was made and whether it was based on gender, race, or other sensitive attributes. Library or digital infrastructure staff are the main actors in this technology deployment pipeline. They should be conscious and reinforce the decision-makers to deploy the technology that includes the specific features for explainability and transparency in the library systems.
What can they do if an institute, library, or repository wants to find out if their website, library catalogue, or other infrastructure they offer is discriminatory? How can they tell who is being discriminated against? Where can they get support or a discrimination check-up done?
First, “check-up” should start by verifying the quality of the data through quantitative and qualitative, mixed experimental methods. In addition, there are several open-access methodologies and tools for fairness check and bias detection/mitigation in several domains. For instance, AI Fairness 360 is an open-source toolkit that helps to examine, report, and mitigate discrimination and bias in machine learning models throughout the AI application lifecycle.
Another useful tool is “Datasheets for datasets”, intended to document the datasets used for training and evaluating machine learning models; this tool is very relevant in developing metadata for library and archive systems, which can be further used for model training.
Overall, everything starts with the right mindset and awareness on approaching the bias challenge in specific domains.
Further Readings
- Artificial intelligence: New Opportunities for Citizen Science, Research and Libraries
- Science Checker: Open Access and Artificial Intelligence Help Verify Claims
- Digital Open Science Tools: How to Achieve More Openness Through an Inclusive Design
- Horizon Report 2020: AI, XR and OER Begin to Catch on in Higher Education
- Open Access: AfricArXiv Facilitates Knowledge Exchange Between Africa and Europe
- DGI Day Practicum: How Does Artificial Intelligence Change the World of Information Professionals?
- Hackathon Coding.Waterkant: How to Improve Library Services Through Chatbots and Artificial Intelligence
We were talking to:
Gunay Kazimzade is a Doctoral Researcher in Artificial Intelligence at the Weizenbaum Institute for the Networked Society in Berlin, where she is currently working with the research group “Criticality of AI-based Systems”. She is also a PhD candidate in Computer Science at the Technical University of Berlin. Her main research directions are gender and racial bias in AI, inclusivity in AI, and AI-enhanced education. She is a TEDx speaker, Presidential Award of Youth winner in Azerbaijan and AI Newcomer Award winner in Germany. Gunay Kazimzade can also be found on Google Scholar, ResearchGate und LinkedIn.
Portrait: Weizenbaum Institute©
View Comments
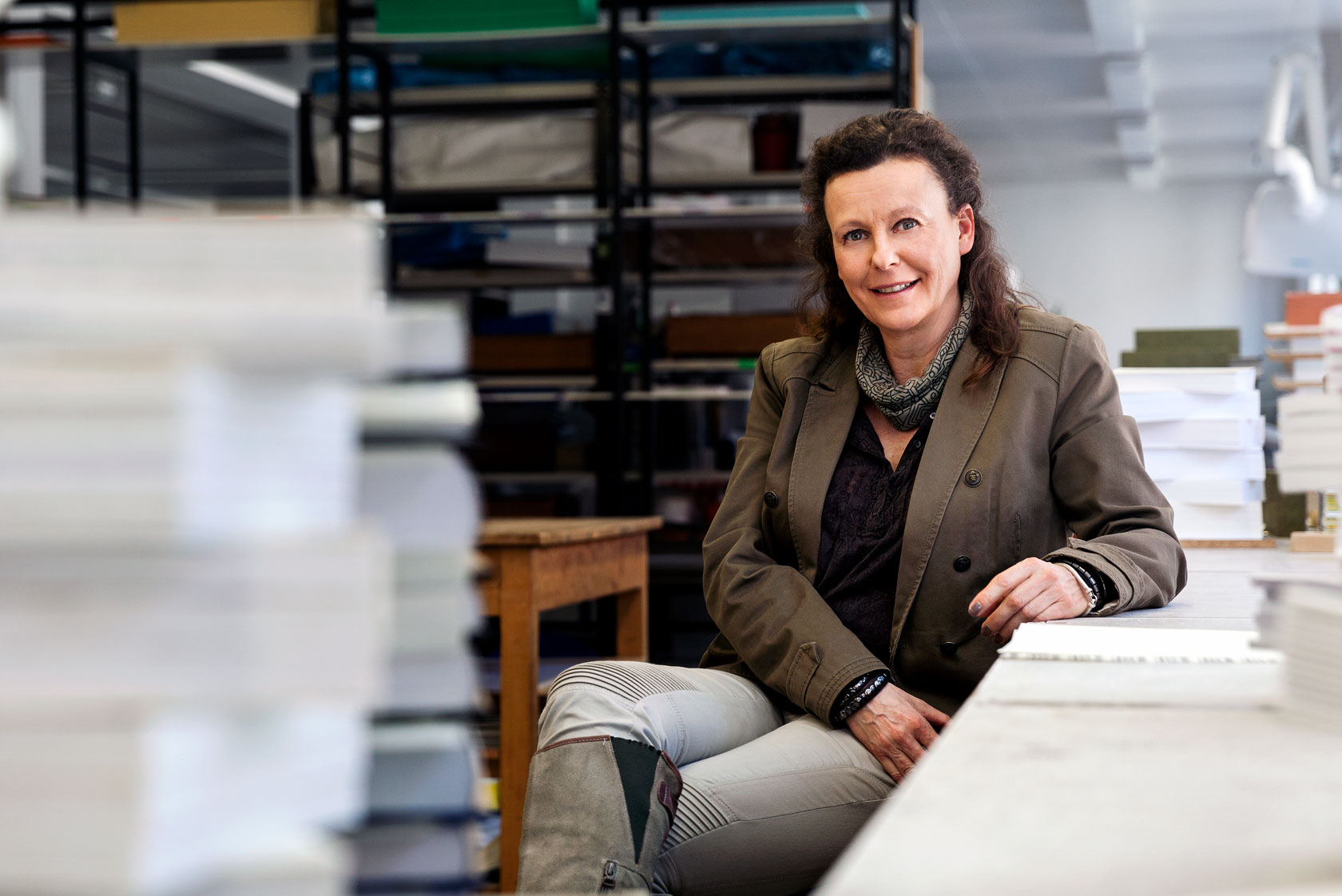
Behind the Scenes: UNESCO Declares Bookbinding as Intangible Cultural Heritage
Many people know about UNESCO's Cultural and Natural Heritage from travelling. Few,...